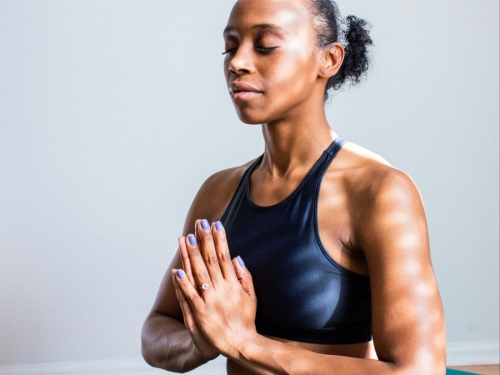
Employee Wellbeing Surveys: What You Need To Know
Discover all you need to know about running an employee wellbeing survey that helps you to achieve your organisation’s goals. Read on for our top tips.
Employee Wellbeing Surveys: What You Need To Know
Discover all you need to know about running an employee wellbeing survey that helps you to achieve your organisation’s goals. Read on for our top tips.
Employee Surveys: What Will They Look Like in 2024?
Employee surveys can be a powerful tool for improving workplace culture and driving your business forward. Find out where to focus your efforts in 2024.
12 Tips For Building An Employee-Centric Listening Strategy
Are you ready to build your employee listening program but want to find out how to put your people in the centre? Read on for our step by step guide.
Employee Engagement & Happiness Platform Review: 10 Software Solutions To Meet Your Needs
Discover our top 10 employee engagement platform software picks, including what we use ourselves to support our people.
Empathetic Leadership: 7 Tips for Being an Empathetic Leader
Progressive organisations are looking beyond traditional strategies for leadership to ensure they can motivate, boost performance and improve the business.
Latest Trends in Employee Engagement
Employee engagement is the most important catalyst for business success. Following these steps will enable a thriving business of the future. Read on.
Ad Hoc Employee Pulse Surveys Vs Always-On Listening
The growing awareness for the need of data on employee's engagement and happiness has lead to a rise in pulse surveys and always-on listening. Read on.
Is Hybrid Working Still The Future?
Conversations about the pros and cons of hybrid working are everywhere. At THI we believe that hybrid working is still the future. Here’s why.
Linking Employee Benefits & Employee Listening
Employee benefits and employee listening work together to help to create a thriving employee culture. Read on to find out how these two elements are linked.
10 Benefits Of Employee Engagement Surveys
There are many benefits of employee engagement surveys. Discover the impact of measuring and understanding your engagement status for yourself.
5 Easy Wins For Your ESG Strategy
Discover our top tips to help you find quick wins within your ESG strategy so that you be successful with ESG in your organisation.
ESG Communication Strategy - Answering Your Questions!
Have questions about building an ESG communication strategy? We have answers! Let’s dive into the details together…